Virtual laboratory opens possibility for machine learning to understand promising class of quantum materials
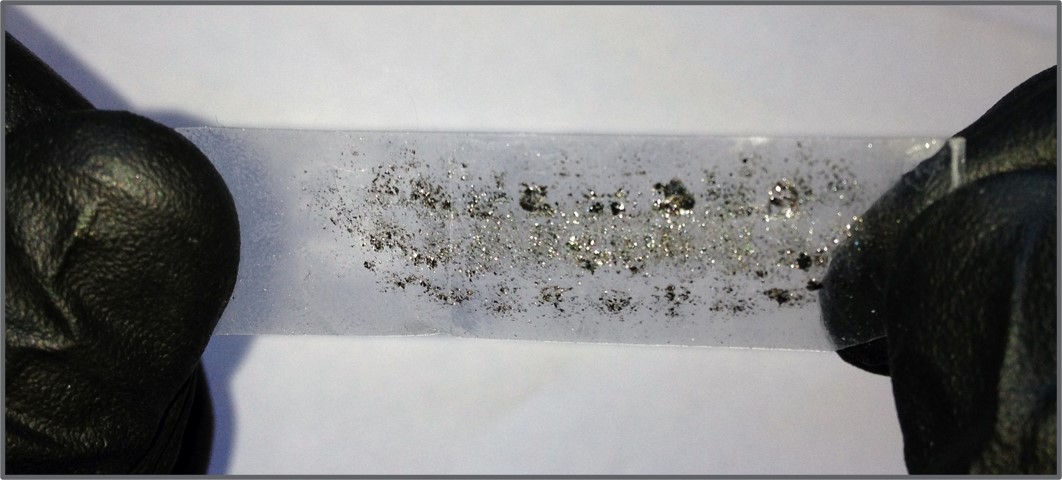
Collecting New Synthesized Quantum Materials: Thomas Edison famously tried hundreds of materials and failed thousands of times before discovering that carbonized cotton thread burned long and bright in an incandescent light bulb. Experiments are often time consuming (Edison’s team spent 14 months) and expensive (the winning combination cost about $850,000 in today’s money).
Expenses and time increase exponentially when developing the quantum materials that will revolutionize modern electronics and computing.
To make quantum material discovery possible, researchers turn to detailed databases as their virtual laboratory. A new database of understudied quantum materials that has been created by researchers at Pacific Northwest National Laboratory (PNNL) provides an avenue to discover new materials that could power gadgets far more powerful than Edison’s lightbulb.
Beyond Edisonian trial and error
Collecting New Synthesized Quantum Materials: “We wanted to understand a general class of materials that have the same crystal structure, but different properties depending on how you combine and grow them,” said materials scientist Tim Pope. This class of materials, known as transition metal dichalcogenides (TMDs), contains thousands of potential combinations, each of which requires a weeks’ long reaction to grow flakes of material the size of glitter.
Making the material is only the first step in understanding what it can do. As PNNL computational scientist Micah Prange said, each flake is “really small, really delicate,” and quantum features will only emerge when studied at super-low temperatures. Essentially, “a whole research program could go into each flake.”
Despite the difficulty of creating and measuring them, each combination holds promise to dramatically improve electronics, batteries, pollution remediation, and quantum computing devices.
Prange said you can think of the flakes as “fancier graphene with a richer phenomenology and more practical possibilities.” Tough, light, and flexible, graphene has been known as the material of the future, with uses in everything from aerospace to wearable electronics.
“The varied properties across this class of materials mean that as we better understand them, one of the combinations could be selected for a desired property and exactly paired to the ideal use,” said Pope, “or even a brand new application.”
Quantum material development of the future
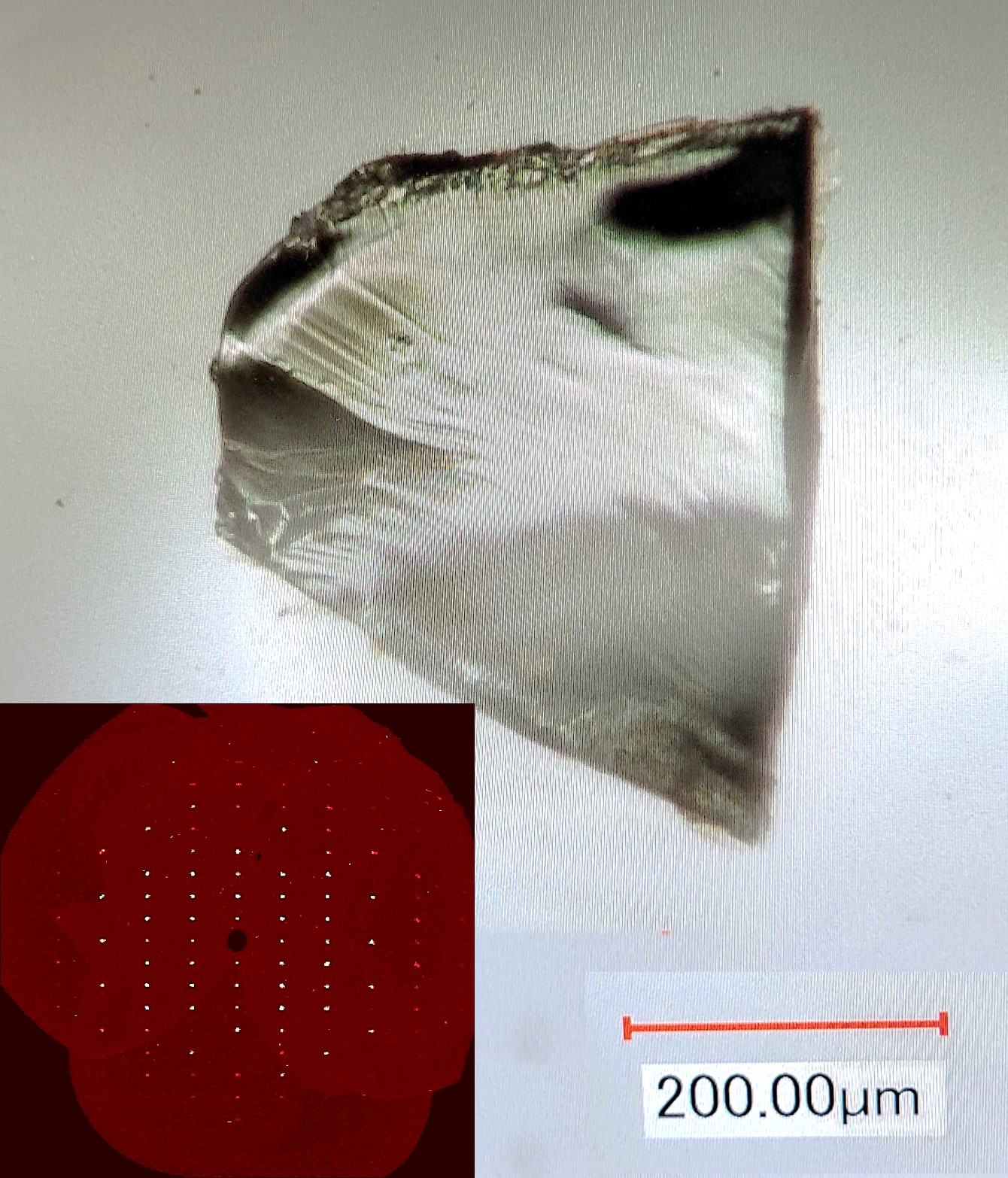
Collecting New Synthesized Quantum Materials: Building the database began with PNNL’s Chemical Dynamics Initiative, an effort to use PNNL’s strength in data science to fill in knowledge gaps left by measurement challenges and experimental limitations.
These particular quantum materials are made by varying proportions of the 38 transition metals, like tungsten or vanadium, in combination with three elements in the sulfur family. They can also be grown in three different crystal structures, meaning there are thousands of potential combinations, all with distinct properties.
Using a type of modeling called density functional theory, the researchers computed the properties of 672 unique structures with a total of 50,337 individual atomic configurations. Before this research, there were fewer than 40 studied configurations, with only a rudimentary understanding of their properties.
“Models can work out the quantum mechanics of how atoms are arranged,” said Prange. “From this, you can say if the material will conduct electricity or be transparent or how hard the material will be to compress or bend.”
Using the database, PNNL’s researchers revealed striking differences in the electrical and magnetic behaviors between different combinations. Importantly, the researchers also found other trends as they varied the transition metal, including a new understanding of transition metal chemistry at the quantum level.
Quantum combinations for machine learning
Collecting New Synthesized Quantum Materials: “When the crystal structure was overlaid with the database, it matched perfectly,” said Pope, speaking of the PNNL-grown flakes that are beginning to validate the modeling results.
“The idea was really to develop a big data set of theoretical simulations so we could use data analytics to understand these materials,” said Prange. “The immediate value of the project is that we did enough different cases to efficiently use machine learning.”
The open-source dataset, published in Nature Publishing Group’s journal ‘Scientific Data,’ offers researchers a strong starting point for exploring relationships between initial structures and corresponding properties. With this information, they can downselect to specific materials for study.
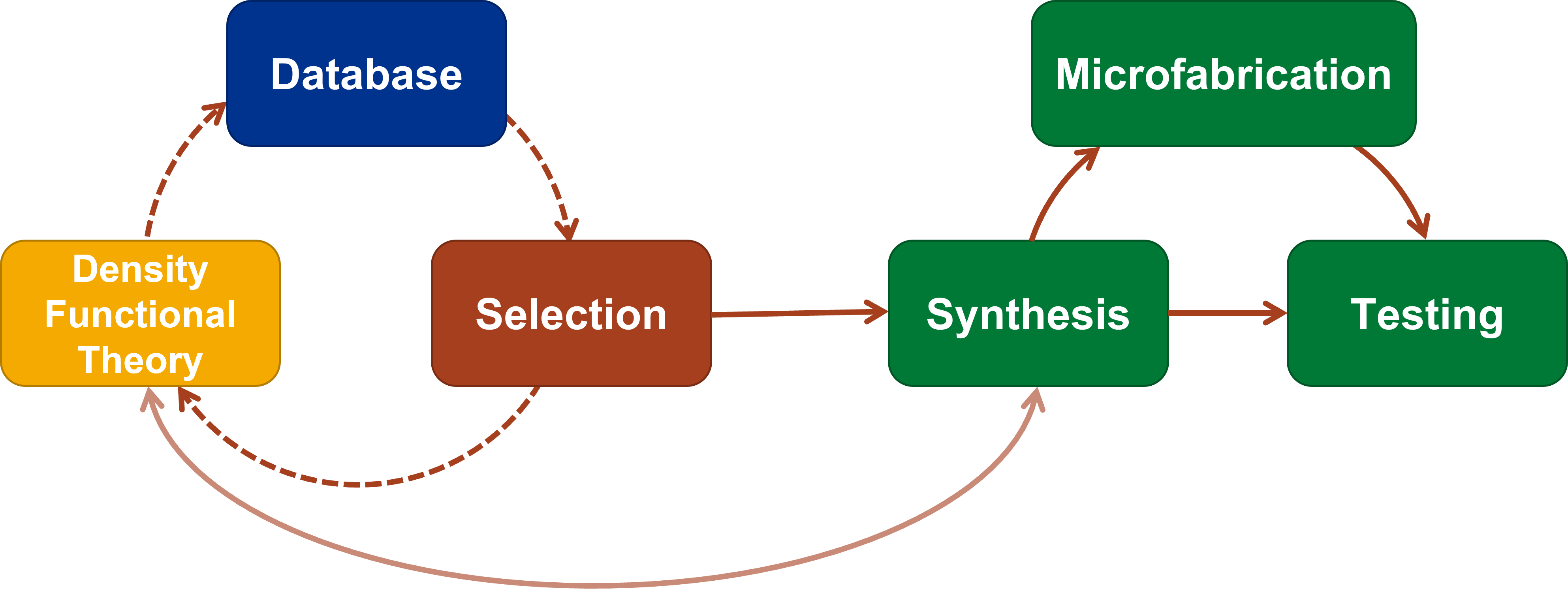
###
About PNNL
Pacific Northwest National Laboratory draws on its distinguishing strengths in chemistry, Earth sciences, biology and data science to advance scientific knowledge and address challenges in sustainable energy and national security. Founded in 1965, PNNL is operated by Battelle for the Department of Energy’s Office of Science, which is the single largest supporter of basic research in the physical sciences in the United States. DOE’s Office of Science is working to address some of the most pressing challenges of our time. For more information, visit https://energy.gov/science. For more information on PNNL, visit PNNL’s News Center. Follow us on Twitter, Facebook, LinkedIn and Instagram.
Collecting New Synthesized Quantum Materials: Original Article
A unique combination of catalysts opens doors to making useful compounds